Generative AI, the technology that allows programs and devices to create new and original content, is rapidly gaining adoption and popularity. Market research firm Gartner predicts that over 80% of enterprise-level firms will have used the technology by 2026. To put things into perspective, this statistic was only 5% in the first months of 2023. This trend is not showing any signs of slowing down, especially as more businesses realize the benefit of using AI. In our post on ‘AI Application Ideas’, we delved into how this technology can take businesses to the next level. Some of the strategies where it can greatly contribute are product demand projections, personalized marketing, and managing talent.
The remarkable progress of generative AI, however, wouldn’t be possible without one crucial element – data.
Data is the lifeblood of generative AI. Just as a painter needs a palette and brush to create a masterpiece, generative AI models require data to learn and produce new content. This data acts as the raw material from which the AI can identify patterns, understand relationships, and ultimately create something new.
With this in mind, take a deeper look into the critical role data plays in making generative AI the cutting-edge software used worldwide today.
The Foundation: Training Generative AI Models
Generative AI foundation models are typically trained via deep learning. Deep learning algorithms are inspired by the structure and function of the human brain, with artificial networks patterned after an interconnected web of neurons. A guide to generative AI at MongoDB explains that these foundation
models are trained on massive datasets. These sources range from text and code to images and audio.
A foundation model that’s trained to understand human language is called a large language model (LLM). A good example is OpenAI and its ChatGPT models, which are trained on text data scraped from books, articles, and code repositories. Another type is a visual foundation model that learns from non-text content to learn how to generate output such as images. StyleGAN, which was introduced by Nvidia, falls under this category. AI models like it can be used to create realistic photos, generate product designs, or even develop completely new artistic styles.
Here’s a simple analogy: imagine you’re training a child to identify different types of dogs. You wouldn’t just show onepicture of a dog. You would show the child photos of dogs of all shapes, sizes, and breeds. Similarly, a generative AI model needs to be exposed to a vast library of dog pictures to learn how to create realistic images of pooches. The more diverse and larger the dataset, the better the model can understand the underlying characteristics of dogs.
Likewise, in a previous post, The Business Blocks showed how to create anime art with AI. The tools for this purpose are built with foundation models trained with datasets of anime content.
Data Quality and Diversity: The Keys to Success
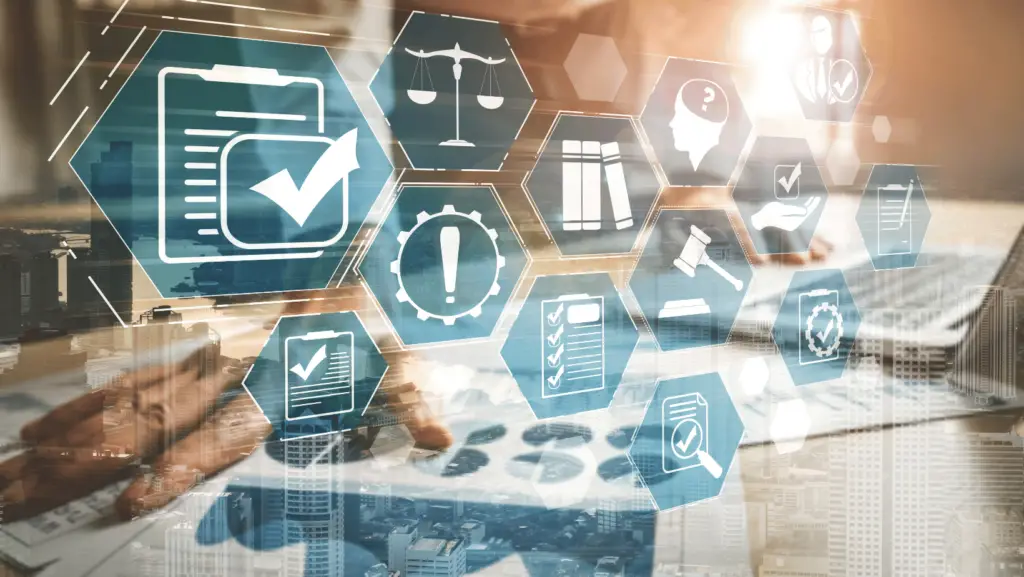
The quality and diversity of data are crucial for the success of generative AI. First off, quality data leads to quality output. If the training data is riddled with inconsistencies, the generative model will inherit those flaws. Take, for example, a model training with poorly written text. Its results will likely have grammatical errors or non-sensical passages.
Moreover, data diversity fuels creativity. A report on AI success at Digitalisation World notes that a diverse dataset exposes the model to a wider range of patterns and variations. All of this highlights the importance of data cleaning and pre-processing before feeding it into a generative model. Techniques like filtering out irrelevant information, correcting errors, and ensuring data consistency are vital steps in ensuring high-quality output.
Data Beyond Training: Fine-Tuning and Creativity
Data’s role goes beyond just training generative AI models, as it also plays a part in fine-tuning the model and influencing its creative direction. Take for instance OpenArt, a generative model trained on massive datasets of artworks. By feeding it additional data specific to a particular artist’s style such as Van Gogh, the model can learn the nuances of the artist’s work and generate images that emulate his style. This allows for a level of control and customization that unlocks new creative possibilities.
Growing with Data: Future of Generative AI
The future of generative AI is inherently linked to the evolution of data. As people and organizations collect and store more data, generative models will have access to even richer datasets. This will lead to greater creativity that can help in everything from generating personalized content to more accurate linguistic responses.
Conclusion
Data is the cornerstone of generative AI’s increasing success. By leveraging high-quality and diverse data, it’s demonstrating the potential to revolutionize various fields and usher in a new era of creativity. For other similarly interesting topics, browse through other posts about AI here on The Business Blocks.